Dotdigital Help Centre
Introducing: New Dotdigital
Discover our new user interface and streamline with unified contacts.
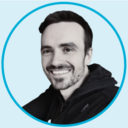
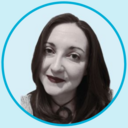
Get started
Get to grips with the fundamentals of Dotdigital.
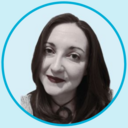
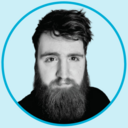
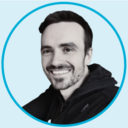
Contacts
Add, edit, and manage your contacts.
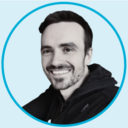
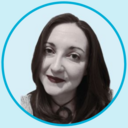
Set up, design, test, and send email campaigns.
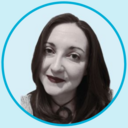
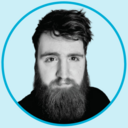
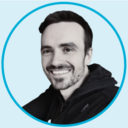
SMS and MMS
Create, test, and send SMS and MMS campaigns.
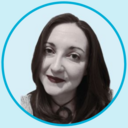
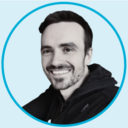
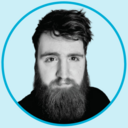
Surveys, pages, and forms
Build custom landing pages, grow your lists, and capture customer information.
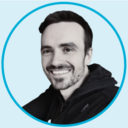
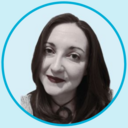
Automation
Automate your workflows and everyday tasks.
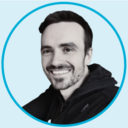
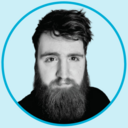
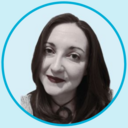
Chat
Offer instant one-to-one support to your customers.
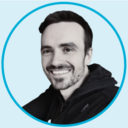
Analytics
Track the performance of your accounts, channels, and commerce.
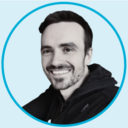
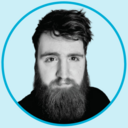
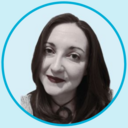
Commerce
Connect your store and grow your marketing around your customers.
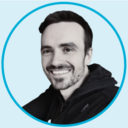
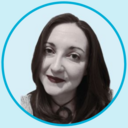
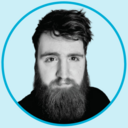
Integrations
Connect with your favourite platforms, stores, and tools using our apps and extensions.
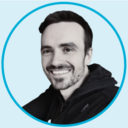
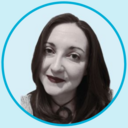
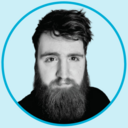
Account settings and admin
Settings, teams, users, billing and payment details.
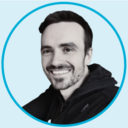
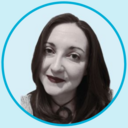
Regulations, privacy, and security
Tools to help you remain compliant.
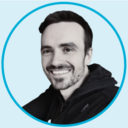
Announcements
Latest updates and new features.
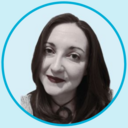
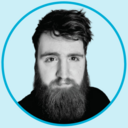
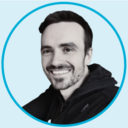